Facial Expression and Emotion Detection in AI Hiring: Unveiling the Truth Behind Candidate Responses
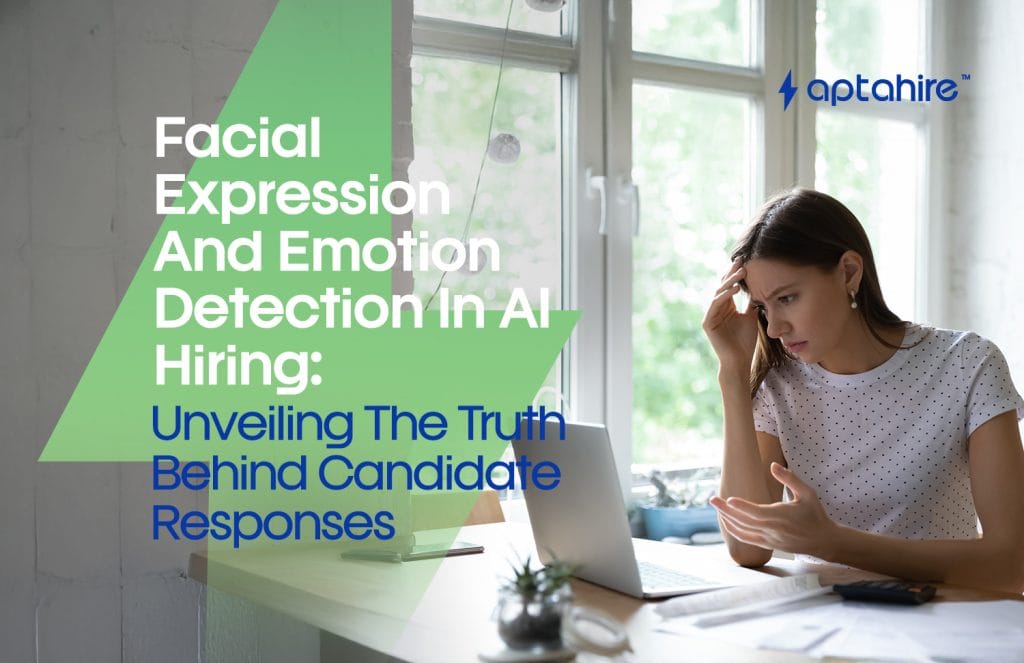
Introduction
Imagine a world where machines can truly “feel”, not in the emotional sense, but by recognizing, interpreting, and responding to human emotions in real time. We’re no longer in the realm of science fiction; this is the promise of Facial Emotion Recognition (FER) powered by Artificial Intelligence. From understanding a patient’s anxiety in a hospital room to detecting a candidate’s stress during a virtual job interview, AI is learning to read the subtle language of facial expressions, micro-movements that often reveal more than words ever could.
In today’s AI-driven ecosystem, emotion has become data. And that data, when analyzed through sophisticated neural networks, opens up endless possibilities for industries ranging from healthcare and education to retail and automotive. But building emotionally intelligent machines isn’t just about feeding images into a deep learning model, it’s about combining psychology, ethics, machine vision, and real-time decision-making into one seamless experience.
In this blog, we’ll break down how FER works, where it’s making an impact, the challenges that come with it, and what developers, businesses, and technologists should keep in mind as they navigate this emerging landscape. Let’s dive deep into the science and soul of emotion analysis through AI.
The Science Behind Facial Emotion Recognition
Understanding Facial Expressions
Human emotions are universally expressed through facial movements, encompassing micro-expressions and subtle muscle shifts. These expressions are categorized into primary emotions such as happiness, sadness, anger, surprise, fear, and disgust. FER systems aim to decode these expressions by analyzing facial features and correlating them with specific emotional states.
Core Components of FER Systems
- Data Acquisition: Capturing facial images or video sequences using cameras or sensors.
- Preprocessing: Enhancing image quality, normalizing lighting conditions, and aligning facial landmarks to ensure consistency.
- Feature Extraction: Identifying critical facial features, such as the curvature of the lips or the furrow of the brow, which are indicative of specific emotions.
- Classification: Employing machine learning models, particularly Convolutional Neural Networks (CNNs), to classify the extracted features into distinct emotional categories.
The Role of Convolutional Neural Networks (CNNs)
CNNs have revolutionized FER by automating feature extraction and classification processes. Their layered architecture allows for the detection of intricate patterns in facial data, enhancing the accuracy of emotion recognition systems.
Applications of FER Across Industries
Healthcare
In therapeutic settings, FER assists clinicians in monitoring patient emotions, facilitating more personalized treatment plans. For instance, AI-driven emotion recognition can aid in detecting early signs of mental health disorders by analyzing patients’ facial expressions during consultations.
Recruitment
Traditional recruitment methods often rely on subjective assessments of candidates. Integrating FER into the hiring process introduces a layer of objectivity by analyzing candidates’ emotional responses during interviews, thereby providing insights into their stress levels, confidence, and overall suitability for the role.
Customer Service
Businesses utilize FER to gauge customer satisfaction in real-time. By analyzing facial expressions during interactions, companies can adjust their approaches to enhance customer experience and address concerns promptly.
Automotive Industry
Driver monitoring systems equipped with FER can detect signs of drowsiness or distraction, alerting drivers and potentially preventing accidents. This application underscores the role of FER in promoting road safety.
Technical Challenges in FER Implementation
Variability in Facial Expressions
Individual differences, cultural variations, and context-specific expressions pose challenges in creating universally accurate FER systems. Models must be trained on diverse datasets to account for this variability.
Real-Time Processing
Achieving low-latency, real-time emotion recognition requires optimized algorithms and robust computational resources, especially when processing high-resolution video streams.
Occlusions and Environmental Factors
Factors such as facial occlusions (e.g., glasses, masks), lighting conditions, and background noise can impede the accuracy of FER systems. Advanced image processing techniques and adaptive algorithms are essential to mitigate these issues.
Ethical Considerations in FER Deployment
Privacy Concerns
The collection and analysis of facial data raise significant privacy issues. Ensuring informed consent and implementing stringent data protection measures are paramount to maintaining user trust.
Bias and Fairness
AI models may inadvertently perpetuate biases present in training data, leading to unfair outcomes. Continuous evaluation and the inclusion of diverse datasets are critical to developing equitable FER systems.
Psychological Impact
The awareness of being monitored can influence individuals’ behavior and emotional expressions, potentially affecting the authenticity of the data collected.
Tips and Best Practices for Developing FER Systems
- Diverse Data Collection: Amass datasets that encompass a wide range of demographics to enhance model generalizability.
- Robust Preprocessing Pipelines: Implement preprocessing steps that address common challenges such as varying lighting conditions and occlusions.
- Regular Model Evaluation: Continuously assess model performance against new data to identify and rectify biases or inaccuracies.
- User-Centric Design: Prioritize user consent and transparency in data handling practices to uphold ethical standards.
Interesting Facts About FER
- Micro-Expressions: These fleeting facial expressions, lasting only fractions of a second, can reveal genuine emotions that individuals might attempt to conceal.
- Cross-Cultural Recognition: While some facial expressions are universal, others are culture-specific, necessitating culturally aware FER models.
- Integration with Other Modalities: Combining FER with voice analysis and physiological signals can enhance emotion recognition accuracy, leading to more comprehensive affective computing systems.
Final Thoughts
Facial Emotion Recognition stands at the confluence of artificial intelligence and human psychology, offering profound insights into human-machine interactions. As FER technology advances, it promises to revolutionize industries by providing deeper understanding and responsiveness to human emotions.
However, navigating the technical challenges and ethical considerations is crucial to harness its full potential responsibly. By adhering to best practices and prioritizing ethical deployment, FER can significantly contribute to creating more empathetic and effective AI systems.
FAQs
1. What is facial emotion recognition through artificial intelligence?
Facial Emotion Recognition (FER) through AI is the use of computer vision and machine learning to identify and analyze human emotions based on facial expressions. The AI system detects facial features and interprets expressions like happiness, anger, sadness, and surprise.
2. Which AI technique is used for emotions and feelings?
Techniques like Convolutional Neural Networks (CNNs), Recurrent Neural Networks (RNNs), and Natural Language Processing (NLP) are commonly used. For facial emotion recognition specifically, deep learning and computer vision techniques are applied to images or video.
3. What is emotion detection by facial expression recognition?
It’s a process where AI systems detect and classify emotions by analyzing facial muscle movements and patterns, such as smiling, frowning, or raised eyebrows. This is typically done using trained models that map expressions to emotional states.
4. What is face detection in artificial intelligence?
Face detection is an AI-based technique that identifies human faces in images or videos. It’s the first step before emotion recognition and involves locating the face region so further analysis (like expression or identity) can be performed.
5. What is the AI that detects emotions?
There are several AI tools like Affectiva, Microsoft Azure Face API, Amazon Rekognition, and FaceReader that specialize in detecting emotions through facial expressions. These tools use deep learning models trained on diverse datasets to interpret emotions accurately.
6. What are the applications of facial expression recognition?
- Healthcare: Monitor patient emotions
- Recruitment: Analyze candidate emotions during interviews
- Education: Assess student engagement
- Retail: Understand customer reactions
- Security: Identify suspicious behavior
- Entertainment: Improve user experiences in games and media
7. What is the primary purpose of emotion detection in AI?
The goal is to enhance human-computer interaction by enabling machines to understand and respond to human emotions. It helps in making AI more empathetic, personalized, and effective in areas like mental health, customer service, and user experience.